Product principles for deploying AI in Vertical SaaS
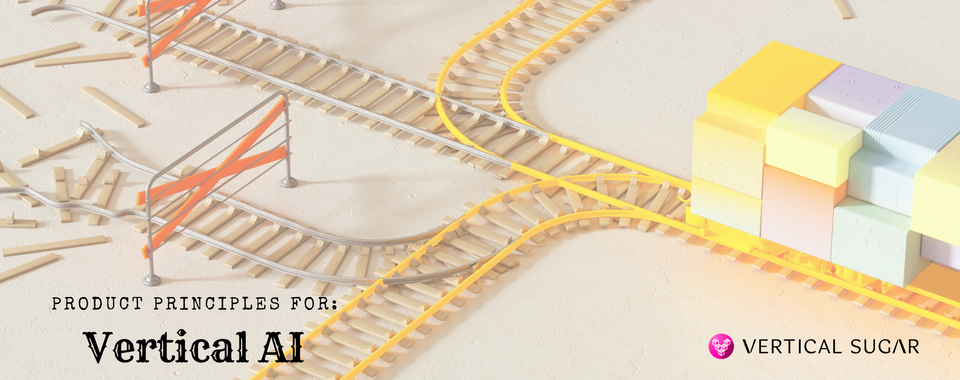
AI is constantly advancing and in certain sub-fields has reached true business viability (e.g, computer vision, data processing, tight domain natural language understanding). However, we are still far from General AI solving generic tasks across business.
The ability to deploy value-added AI is tightly linked to how much you can constrain the domain where it operates. For example: designing a general chatbot for service is still far from feasible. However, a chatbot for barbershop scheduling support is very doable and not even that hard. General computer vision to understand any image and it’s meaning? Very hard. However, AI that can count livestock is already available.
It’s now going to be up to the Vertical Software providers to decide where and how to deploy AI to help their customers.
We’ve put together our starting list of principles and will add to it over time.
Product management principles for deploying AI into Vertical SaaS
A. Start with Business-Value to Tech
The good old product management principles still apply - start with defining high business value uses cases, make a long list, since we will soon deprioritize many. Once you’ve done that, answer the following. Back-of-the-envelope answers are enough for this stage:
- Define the impact and metrics you will measure: cost reduction, effort reduction, error reduction, speed improvements, etc. can be almost anything, but if it’s hard to very clearly it’s an indication that this may not be the right place to start.
- Define how you will measure the metrics - this is a deep dive on #1 to make sure we will be able to measure and then optimize. AI deployments can start out with poor results and rapidly improve to amazing results, but only if you are able to measure and adjust towards your goals
- Training Data - next ask yourself questions about your current access and organization of data. Generally, the more organized data you have about the issue you want to impact and faster and better results you can expect.
B. Now explore what's out there: Tech-to-business Business-Value
It’s now time to explore and understand what’s out there. The world of AI is rapidly evolving so ongoing research is required. A tight google search is a way to start, but also using services like GLG, Alpha sites, and others to find domain experts is highly suggested.
Start with looking for specific industry solutions for problems you identified above: You’d be surprised how often startups or university research teams pick a topic in your domain. Finding a tailored solution could be fantastic for next steps, however it’s critical to understand the business viability of the provider of the solution as it will impact how you proceed. If for example you found a university team that built a solution, you need to consider that the true R&D will still fall on your internal teams. Even if you don’t deploy their tech, you should include them as consultants in some way.
Next go horizontal - being a vertical saas player we should not invest the massive R&D effort required to build AI solutions from the ground up. So now it’s time to understand what horizontal tools are relevant for our needs, and how many of our high value use cases can they solve. If you are early in your journey, I suggest prioritizing time-to-value over other considerations. That is: how quickly can you build, train and deploy your first high value use case. AI is a journey, small early success pave the road for future bigger investments.
Find a couple of expert advisors - now that you have an initial sense of what’s going on in AI and specifically AI in your vertical it’s time to have a couple of experts in your corner. You don’t need much but also don’t want to go at it alone. A small advisory team can be highly beneficial in reviewing and providing direction which will save a lot of time and improve results down the line.
C. Finally down-select to define and execute a proof-of-concept
This is an iterative journey and it’s critical to learn by doing, so the goal should be getting to doing.
- Down-select by time-to-value, price and likelihood of success. Prefer shorter time frames (weeks or a low number of months) to get to results. Even if the impact is lower.
- Impact must be clearly measurable. Agree on the metrics and how you will measure them. Together with vendors and advisors define what is a reasonable result to get to quickly, and how rapidly it should improve once deployed.
- This is not your typical feature deployments - the process is different, an “AI feature” isn’t ready when dev is done, it’s ready when the metrics say so and you see the results.
Once you’ve successfully executed your first 1-2 proofs of concepts, you’ll be in a great place to evolve to the above process for your team and vertical.
We're a startup, if you found this helpful share with a colleague or reach out to us to let us know (just hit reply). We'd love to hear from you.
Stay Vertical!
Suga